Development of a robust technique for estimating extracellular free water and neurite density from single-shell diffusion MRI data
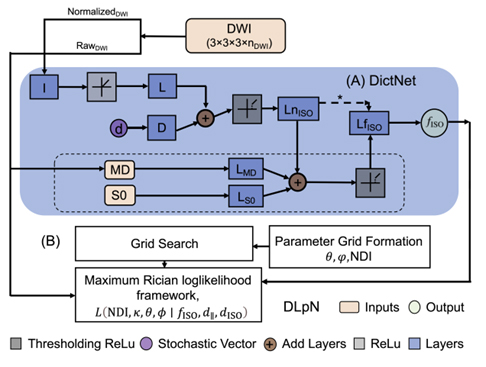
Figure 3a: Schematic of the proposed Dictionary-based Learning prior NODDI (DLpN). The stochastic dictionary learning framework (DictNet) estimates fISO prior and then used with NODDI Rician log-likelihood estimation steps for NDI and κ mapping. A) DictNet, B) DLpN.
The extracellular free water (FW) signal fraction, indicating the relative amount of freely diffusing water in the extracellular space, can unveil neuroaxonal damage impacting tissue diffusion characteristics. FW correlates with neuroinflammation and neurodegeneration in various disorders, including HIV, confirmed through PET studies measuring microglial activation via TSPO. Existing FW estimation techniques using free water imaging rely on diffusion tensor imaging (DTI), limiting their ability to characterize tissue microstructure comprehensively, unlike the neurite orientation and density imaging (NODDI) model.
We recently developed NODDI parameter maps with single-shell data (i.e., b=1000 s/mm²), utilizing FW as a prior. This approach, independent of the NODDI model constraint, employs a stochastic sparse dictionary-based network (DictNet) to predict FW, trained on in-vivo and simulated diffusion MRI data. Using the known FW, the NODDI framework estimates the neurite density index (NDI) and orientation dispersion index (ODI). Our method outperforms other approaches in synthetic data simulations and in-vivo HCP data, surpassing original NODDI, and other advanced approaches. However, recent research identified compartment-wise T2 and echo time (TE) dependency in NDI and FW. To address this, we propose incorporating voxel-wise T2 values into our model and optimizing diffusion gradient directions and b-values. This study aims to develop and validate a robust, time-efficient technique for investigating neuroinflammation and neurodegeneration in the clinical population.

Figure 3b: A) NODDI parameters ( fISO,ODI,NDI) in combined HSV colorspace. B and C illustrates the color-map depiction.